For the last couple of weeks I have been actively job hunting and in one of the place I interviewed I was asked if I can do take-home assignment in Go. Since I had already mentioned in the interview I had been learning Go on the side. I agreeded finishing the assignment in Go thinking this might be a good challenge to take on and by doing this I might have a chance to showcase my ability of picking up new things quickly.
Plus having a deadline to complete the take-home assignment in a week could also accelerate my Go learning, as it provides a time based goal to work towards.
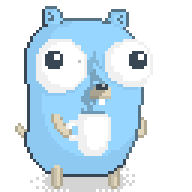
So for a week I spend most of my time reading parts of Learning Go, refering to tutorials Learn Go with Tests, watching old episodes from justforfunc: Programming in Go, a lot of stackoverflowing and talking to friends who use Go as primary language at work to get some pointers(no pun intended) on best practices, blogs to follow etc.
After a week, I finished writing gogrep, a command line program written in Go that implements Unix grep like functionality.
gogrep has a fairly small subset of features it can search a pattern from a file or a directory and also has support for flags like
-i
: Make the seach case sensitive.
-c
: Count number of matches.
-o
: Store the search results in a file.
-B
: Print 'n' lines before the match.
While adding these features I also added a fair bit of tests to the project to see how tests are written in Go.
At the moment the test coverage of the entire project is around 72.0%
where all the major packages that server as helper or have buisness logic have a coverage greater than 83%
and a very few have 100%
.
Since gogrep is my first Go project, I do have some loose opinions about the language and the tools it offer. Primarly from the prespective of Pythonista, who has been using Python as a primarly language for the last four years.
Go does not have classes and it's probably good: If you are coming from a background of classes and methods, you can relate some of it with receiver functions. Receiver functions come very close to behaving like methods for a class. Having written a few receiver functions to for a struct the straight forward use case becomes a no brainer but it might get a bit complicated once you start refactoring a code the second or the third time.
Poniter! Oh my! : If you have every done pointer airthmetic in C/C++ you know the pain. Thank god in Go you don't have to deal with pointer airthmetic. You still have to be careful since you are dealing with memory addresses and it can get complicated but it's defenitely not that bad. You get use to it.
Tooling: I coded the entire project in VSCode and I loved the Go support it has, go-static check suggestion for code snippetes are quite helpful and for tests automatically changing a color of the test file to red at that instant when the associated function is changed, the whole Developer Experience is amazing. I have heard even better things for GoLand by Jetbrains from folks who write Go daily, can't wait to try that out. Besides that gofmt
, golint
, go vet
and go test
were some other things that I found to be really handy. I did not play around much with the debugger so can't comment much on that.
Makefile to the resuce: Since go gives you a single binary in the end you have build it every time to check you code changes. Having some automated way like a Makefile
makes things really easy. So I would suggest during your initial project setup do invest in making a good automated process for building and checking your changes.
Error handeling pattern: Go has this pattern for handling errors which is very straight forward but sometimes it might seem a bit too much in terms of repetitve code.
if err != nil {
// Do something here to log/return error
}
This seems to be the only way to handle error at least that I know of. So most of my code was be sprinkled with a lot of these statements if err != nil
in the top layer of the abstraction, for gogrep it was the main.go
when I was calling func ParseFlags()
for processing arguments.
Like
// main.go
conf, output, err := parseflag.ParseFlags(os.Args[0], os.Args[1:])
if err == flag.ErrHelp {
// Print the usage of the CLI
fmt.Println(output)
os.Exit(1)
} else if err != nil {
fmt.Println("Error: \n", err)
os.Exit(1)
}
//parseflag.go
func ParseFlags(searchWord string, args []string) (config *Config, output string, err error) {
// Supressed code
err = flags.Parse(args)
if err != nil {
return nil, buf.String(), err
}
// Supressed code
if len(flags.Args()) == 0 {
return nil, "", fmt.Errorf("missing argument searchword and filename")
}
// Supressed code
return &conf, buf.String(), nil
}
- Types magic: Since Go is statically typed that means when defenining the variable I have an option to either just declare it with a type and have a zero value in it or directly initializing it with some value.
Even with functions I had to write a signature of input types and output.
func ParseFlags(searchWord string, args []string) (config *Config, output string, err error)
Having types in the function signature and output became such a great advantage later on both developer experience when refactoring and re-reding code that I wrote days back.
I became a fan of types in the codebase to such an extent that now I am all in for type annotations in my Python code if they can give me similar advantages.
- Things I skipped: One week is a very small time to peak into a language features. So obviously I skipped a lot of Go features too like interfaces(I might have used them like io reader but for sure haven't written them), generics and defenitely did not touch goroutines and channels. One thing that most of the people praise Go for. There might be more but from a birds eye view I can not think anything else.
Overall, it was really fun week of learning something new and not using something that is already in my toolkit.
If I do more Go project in the future I would defenitely like to do a talk “Go for Python Developers” where I talk about my experinces of building a series of projects in both Go and Python like:
CLI tool
A key value store
A fully featured backend system for ecommerce website/twitter clone/blogging engine that will include REST APIs, relational DB, Redis for caching, RabbitMQ as a queue, async workers for processing, etc. As close I can get to the real thing.
Thus giving out more stronger opinions that I can back and giving a much deeper overview on what to expect when working with both the languages.
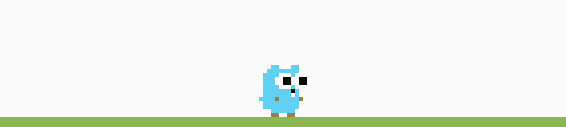
References:
– Gopher Gifs: Images